Fast, scalable, clean, and cheap enough
How off-grid solar microgrids can power the AI raceDecember 2024James McWalter (Paces), Nan Ransohoff (Stripe)
Thank you to many for providing feedback on previous drafts: James Bradbury (Anthropic), David Robertson (Tesla), Austin Vernon (Orca), Alec Stapp (Institute for Progress), Casey Handmer (Terraform), Jesse Peltan (The Abundance Institute), Shayle Kann (EIP), and Andy Lubershane (EIP), James McGinniss (David Energy), Allison Bates Wannop (DER Task Force), Adam Forni
Summary
Context
- Hyperscalers need enormous amounts of power ASAP to power AI datacenters. While highly speculative, estimates range from ~30-300 GW by ~2030.
- Speed is paramount. Procuring huge amounts of energy fast is a strategic imperative for hyperscalers and is of national security importance as well.
- Grid expansion today in the US is slow. While emerging clean firm generation technologies may promise eventual integration with datacenters, these technologies are unlikely to scale fast enough to meet enormous near-term datacenter energy needs. Many believe the AI needs in the US will most likely be met by building colocated natural gas power plants.
- While climate is not the top priority of hyperscalers today, building more natural gas would further increase emissions, which the US is trying to reduce. It would be preferable if we could find ways to build more energy without emitting more, without sacrificing speed or meaningfully increasing cost.
Key questions
- Could off-grid solar microgrids in the US be big enough, fast enough, and cheap enough to be a compelling near-term alternative to building more natural gas power plants to meet near-term AI energy needs?
- If yes, what would that look like and how would one do it?
What we did
- Scale Microgrids ran 20-year powerflow models for thousands of site configurations supporting a 100-1000 MW 24/7 load and ran them them all through Lazard's LCOE model1 to find the best performers. Paces then did a search for all the land in the US Southwest that could accommodate them (filtering for things like distance from natural gas pipelines, property owner type, minimum parcel size, etc.).
- Download the full LCOE workbook developed by Scale Microgrids to play with assumptions.
Key findings
- Off-grid solar microgrids are categorically faster than new grid interconnections (5+year queues) as well as off-grid colocated gas turbines (3+ year lead times).
- Estimated time to operation for a large off-grid solar microgrid could be around 2 years (1-2 years for site acquisition and permitting plus 1-2 years for site buildout), though there’s no obvious reason why this couldn’t be done faster by very motivated and competent builders.
- While solar scenarios modeled in this paper also include 125% capacity of gas engines, these are easier to acquire and faster to build than large dedicated, always-on gas turbines.
- Rental generators—such as those being used by XAI prior to getting a grid connection—are potentially the fastest ‘absolute’ path to power but their limited availability would be quickly exhausted by large-scale adoption (in addition to their high costs at >$300/Mwh). These can be utilized before a final project is commissioned for either off-grid solar microgrids or colocated gas turbine approaches.
- Off-grid solar microgrids today are near cost parity with natural gas and cheaper than other clean alternatives. Opportunities for further cost reduction are significant.
- A microgrid that supplies 44% of lifetime demand from solar and includes 125% natural gas backup is approximately the same cost as using large off-grid natural gas turbines ($93/MWh versus $86/MWh).
- A system supplying 90% of lifetime demand from solar is cheaper than repowering Three Mile Island ($109/MWh versus $130/MWh).
- Further power system design optimization enabled by off-grid solar could reduce cost by another 10%+.
- Off-grid solar microgrids are enormously scalable, with >1,200 GW of datacenter potential in the US southwest alone.
- This is enough suitable land to cover ~4-40X all of the datacenter growth projected in the US through 2030 (~30 to 300 GW of new development by 2030).
- The vast majority of this is in West Texas, which is mostly a function of gas pipeline density (if you relax this constraint by using diesel backup generators instead, you can build almost anywhere with good sun). And, >95% of the land is private, making it commercially viable today.
- Paces has a database of exact parcels that meet these criteria with contact information for landowners. Further design optimizations could further reduce land requirements by ~25%.
- Between 0.4 billion tons (30 GW new datacenters) and 4.1 billion tons (300 GW new datacenters) of CO₂ emissions could be avoided between now and 2030 if every new AI datacenter was built using the 90% solar configuration. At approximately $50/ton CO₂ reduced, replacing gas-powered datacenters with off-grid solar microgrids represents a cost-effective emissions mitigation strategy.
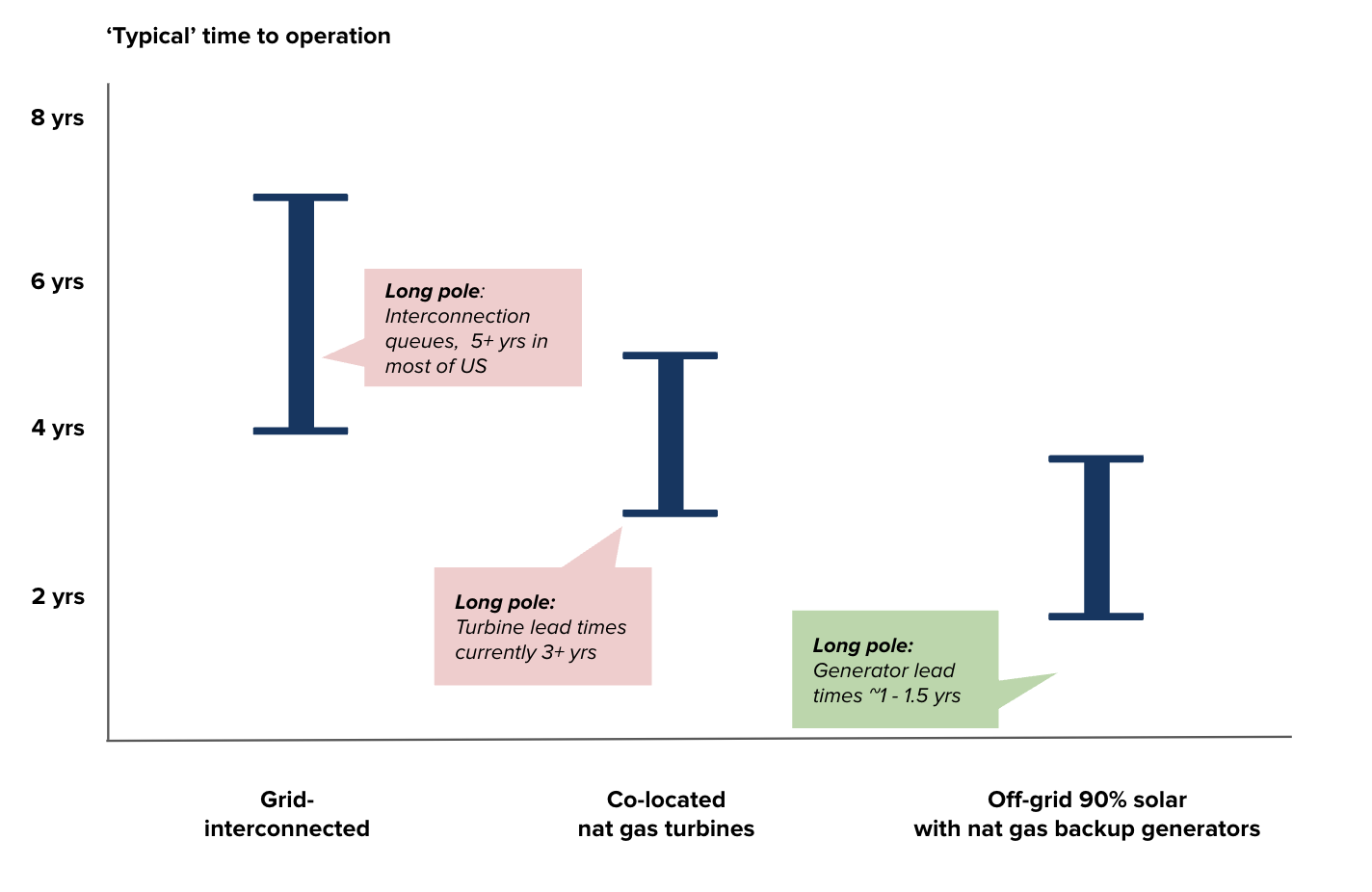
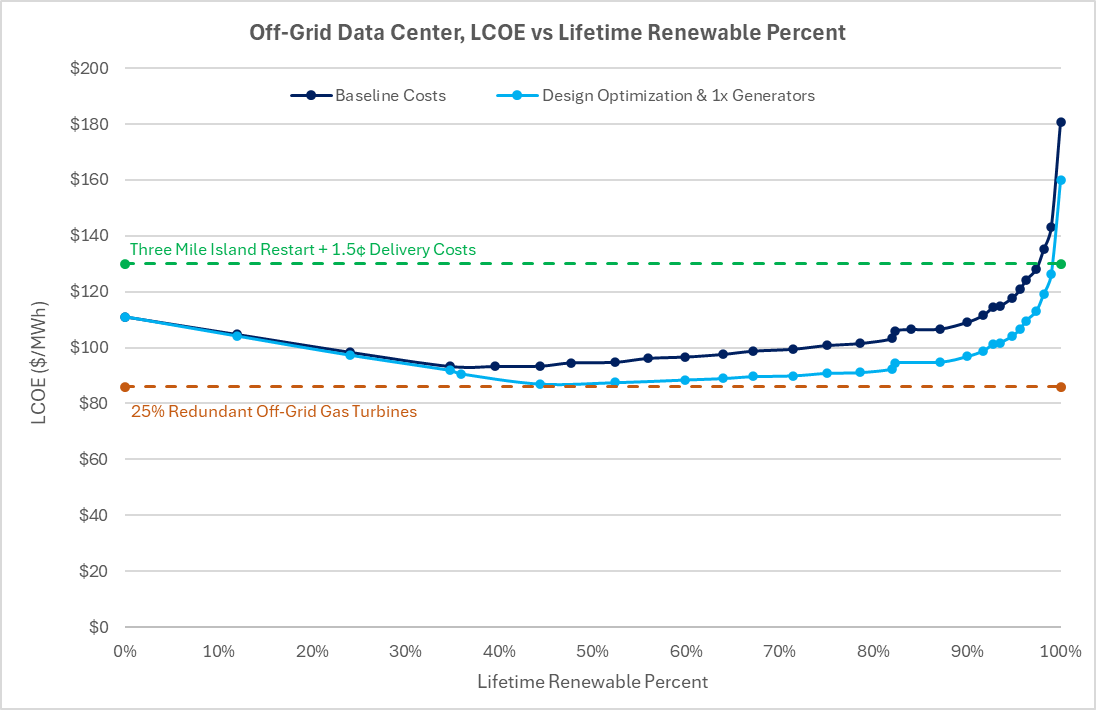
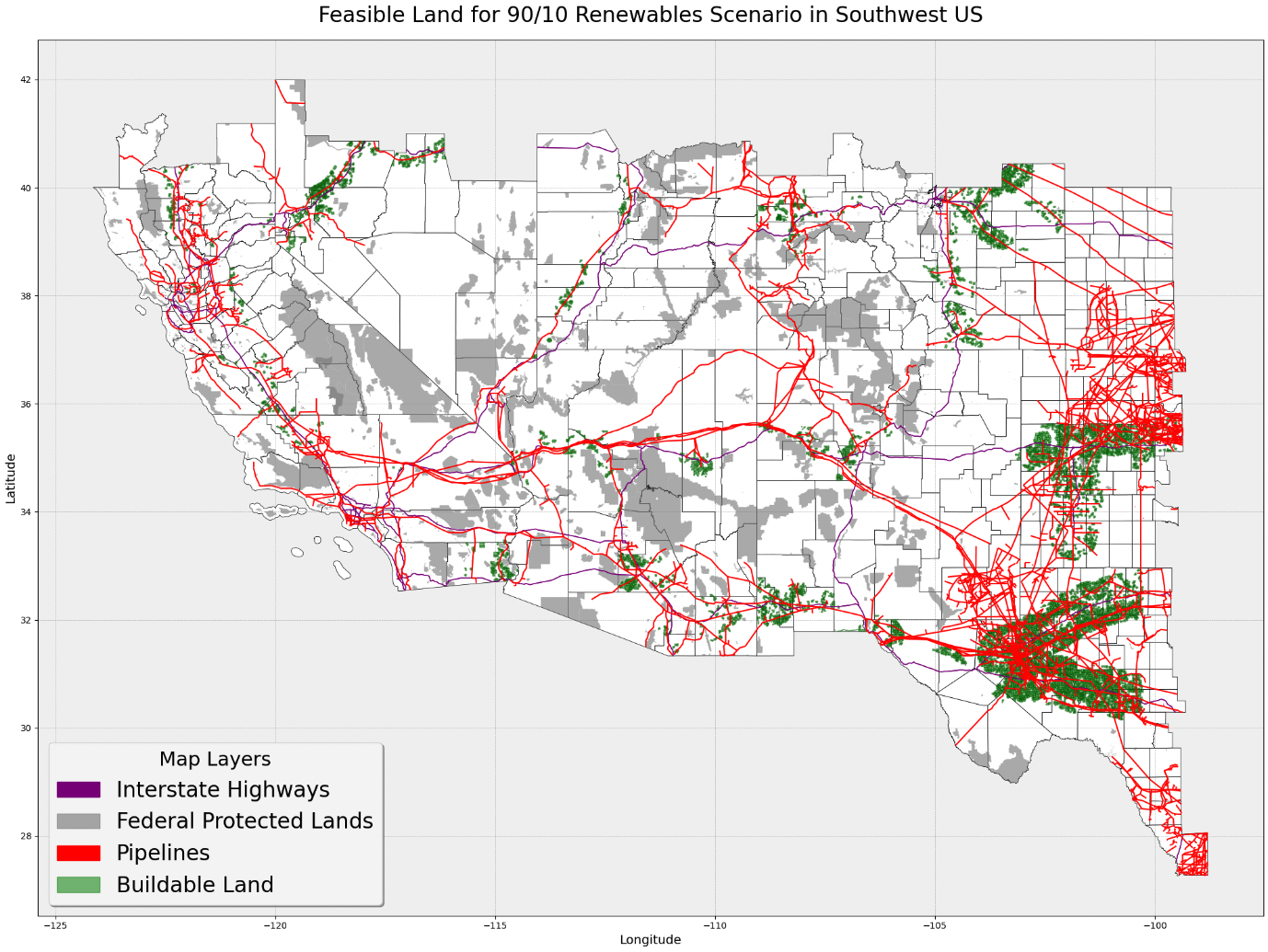
What do hyperscalers care about?
The goal is to maximize absolute compute ASAP, which means accessing huge amounts of energy ASAP. While all else equal they’d prefer cheap and clean, they first and foremost care about scale and speed.
Datacenters for inference vs training have different energy requirements. Inference requires proximity to end-users and a high degree of reliability and redundancy in energy supply. Training datacenters are more geographically flexible and can tolerate less redundancy, though high GPU costs still penalize any significant downtime.
The main focus in this paper is training datacenters since the requirements are less stringent and allow for flexibility in locating compute in areas with high solar potential and cheap land. Estimates vary, but training datacenters likely represent about half of new capacity expected by 2030.
Our understanding of key requirements and rough prioritization is summarized below. Of course, they may differ in type or priority by user.
Criteria Priority Requirement Scale
P0
~30-300 GW total by 2030, ~50% training/~50% inference. Triangulating from both hyperscaler interviews and:
- Jesse Jenkins (Princeton): ~20 GW
- Goldman Sachs: ~30 GW
- International Energy Agency: ~40 GW (global growth, not US specific)
- McKinsey: ~50 GW
- Situational Awareness: ~400 GW is the upper end of anything we’ve seen
Minimum site size: 100 MW capacity on contiguous land.
Minimum cluster: 500 MW total capacity within 10 miles. This could be composed of several smaller sites in the same geographic cluster or one very large site.
Speed P0 ASAP. Speed is relative to whatever the best alternative is. The perceived fastest option right now is colocated natural gas when grid connections/capacity are not readily available. Reliability P1 Approximately 125% of capacity (optimize total cost where you assume downtime translates 1:1 into chips) Cost P1 Generally aim for <$100 per MWh. Energy costs are only a portion of total datacenter costs (generally less than 50%), and energy costs have been rising in recent years as demand has increased. A good reference point is the average price for industrial electricity in the US—a bit over $80/MWh. Risk P1 Time risk and cost risk. In evaluating options, buyers will consider outcome predictability vs the next best alternative. Effort/complexity P1 AI is complicated enough as is, adding on additional complexity is not desirable. That said, many of these companies already have teams that can deal with additional effort/complexity so long as they can still be confident the total time to power is at least as good as the next best alternative. Emissions intensity P2 Nice to have, but won’t sacrifice speed or scale for it. If they can do both at the same time, great, but everything is secondary to speed. Still, most major tech companies have strong climate commitments, and there is some risk to the long-term social licence of AI if emissions concerns remain unaddressed.
What are the current options?
What are the current options for meeting AI energy needs?
- Expand the grid. The problem is that this takes a long time and is expensive.
- Interconnecting large new sources of electricity is taking longer and longer, a median of 5 years for projects built in 2023.
- The cost of delivering (not generating) power via the grid is growing meaningfully faster than inflation – about 65% over a ten year period.
- Siting large new electricity users (datacenters, reindustrialization, EV charging hubs, etc.) has also become very difficult, with operators reporting wait times of many years.2
- This is not surprising, given the institutions that manage the power system have not had to service new electricity demand for nearly 20+ years. In the face of rapidly growing demand from AI datacenters, industrial on-shoring, and electrification, the grid is short generation and network capacity. This will likely be the case for the foreseeable future.
- Restart mothballed facilities like Three Mile Island. The problem is there’s a limited number of these opportunities.
- There are relatively few recently decommissioned nuclear power plants that could be cost-effectively and quickly repowered.
- While repowering shuttered coal plants could be an option in some locations, both local air pollution and greenhouse gas emissions make this problematic for hyperscalers. It also likely is not the fastest option if the plant has been closed for more than a few years.
- Build off-grid, colocated clean-firm energy like geothermal or new nuclear facilities. These are unlikely to get built at the speed and scale necessary to meet near-term AI energy needs.
- In the medium to long-run, clean firm power — possibly off-grid/colocated — may represent a low-cost zero-carbon approach for powering datacenters at huge scale.
- Prior to 2030, enhanced geothermal could fulfill some of the scale needed. For example, Fervo Energy is building 400 MW of enhanced geothermal in Utah that is expected to be online by 2028.
- Still, clean firm solutions scaling to 30 GW+ by 2030 is very unlikely.
- Build new datacenters and new energy infrastructure next to existing utility-scale solar and wind. However, the opportunity here is limited as few utilities have excess existing clean energy capacity that is not currently being utilized.
- There was ~250 GWs of existing utility-scale solar and wind generation capacity in the US by the end of 2023 and recent announcements indicate big tech companies may be pursuing a strategy of locating new datacenters next to these assets.
- Although difficult to estimate, the vast majority of these generation assets have existing offtake agreements, making financial restructuring difficult. The true quantity of viable sites is likely significantly lower than new datacenter capacity needs.
- Furthermore, using energy originally intended for the general public to power AI datacenters may be met with community or even federal pushback.
- Power datacenters with rented, portable gas/diesel generators until permanent power can be secured. This is a reasonable stop-gap solution to get power fast, though the scalability of this strategy is currently limited.
- This option can apply to any of the above options. For example, the xAI site in Memphis is running on rented portable gas turbines while they wait for the existing site grid infrastructure to be upgraded. There are a few challenges here:
- (1) They have discovered training loads require a battery buffer to maintain power quality, and few companies other than Elon’s have access to on demand utility-scale battery storage equipment to pair with rental power.
- (2) Relying on this approach for all planned US datacenters would quickly overwhelm the available fleet of large-scale rental generation.
- (3) Most users of rental power plan to transition once possible because this approach carries very high costs and generally reliability is lower than permanent infrastructure.
- In short, this is probably not a very generalizable approach, though for those who can do it, it can be a useful stop-gap for most of the solutions above, including off-grid solar microgrids (to be discussed shortly).
- Build off-grid, colocated natural gas. Many groups we spoke to consider this to be the most viable near-term option.
- It’s often the fastest option to get a new datacenter built in areas lacking existing grid capacity.
- However, there has been limited experience to-date with large off-grid gas turbine plants, and the rapidly increasing demand for gas generation may result in significant delays for acquiring some equipment—especially turbines which are reported to currently have 3+ year lead times.
Off-grid solar microgrids have been conspicuously absent from most hyperscalers’ plans, which is surprising given they are likely the only clean solution that could also achieve the scale and speed requirements described. We wanted to know whether off-grid solar microgrids could meet the needs of hyperscalers—and specifically if they could be a competitive alternative to just building more off-grid natural gas. That is the subject of the rest of the paper.
The case for off-grid solar microgrids
We’ll walk through our findings in four parts:
- Cost: How much would this cost?
- Scale: Is there enough accessible, buildable land to power the near-term AI race? Where?
- Speed: How fast could these be built?
- Climate: What would the emissions impact be?
Cost: How much would this cost?
We’ll first describe what we did and then describe the findings. What we did:
We developed an off-grid powerflow model to examine system performance across 20 year time lines for any datacenter size and location. We ran this model for about ten-thousand iterations across selected 100 MW sites (clusterable to 500 MW) in the southwest to find the ideal system configurations (solar, battery, and generator capacity) for a variety of costs and renewables percentages. All solutions considered either 125 or 100 MW of standby generation. This part was done by Scale Microgrids, who has deep expertise in modeling and building behind-the-meter power systems.
The outputs of this model were then run through a Levelized Cost of Electricity (LCOE) model to understand the costs of the solution space and identify the best performers. The LCOE model was developed to mirror the methodology used by the well known Lazard report, with the key difference being that this is a full system LCOE, which means all costs necessary to operate this power system year-round were considered. The model utilizes capex, opex, equity, debt, depreciation, taxes, tax credits, etc. and solves for the 20-year price that generates the specified equity returns. Note that the model incorporates eligible solar and battery costs receiving the 30% investment tax credit. However, many areas throughout the southwest qualify for the 10% energy communities adder as well. Download the full LCOE workbook developed by Scale Microgrids to play with assumptions.
Cost details: All industry standard costs were used for solar PV, battery, generator, and system integration. For the solar portion of the design, bifacial panels, a 1.2 ILR, and single-axis trackers at typical GCRs were specified – all very standard for a solar system of this size. For batteries, the Tesla Megapack 2XL (4-hour version) was used as the reference design because it is a well known product with publicly available data. There is less publicly available data on natural gas generator costs, but Scale used typical industry numbers for prime-power capable systems and specified 1.25x capacity relative to load. Lastly, the system integration cost is least common, since there are few examples of off-grid power systems this large, so Scale estimated these independently. Below are the unit costs used in the base case of the LCOE model.
We also ran an off-grid gas turbine reference case through the same LCOE model, using similar industry standard costs. The gas turbine sizes, costs, and efficiencies were assumed to be similar to those currently used at the xAI site. This reference case also included 1.25x capacity, since turbines running 24/7 off-grid will go down at some point for maintenance.
What we found:
There’s a compelling set of possible site configurations LCOE of $93/MWh (44% renewable) to $109/MWh (90% renewable). While costs increase steeply as the portion of renewable generation approaches 100%, costs are quite competitive up to around 90% of lifetime hourly energy demand met by solar+storage.3
For nearly the same LCOE as an off-grid natural gas turbine ($86/MWh for gas vs $93/MWh for solar+storage+generators), you can get a microgrid that’s 44% renewable. The relatively low premium here reflects just how cheap solar and batteries have become, and its advantages in displacing expensive gas consumption. We’ll call this Scenario 1, our cheapest scenario/most similar to natural gas in cost.
For a significantly lower LCOE than the Three Mile Island nuclear reactor restart + minor delivery charges ($109/MWh versus $130/MWh), you can get a microgrid that is 90% renewable. We’ll call this Scenario 3, which is the most expensive, but we know this price is acceptable at least to some because it’s what Microsoft is paying per kWh for power coming off of Three Mile Island.
An intermediate case with 82% renewables, comes in at $103/MWh. We’ll call this Scenario 2.
There are real reasons solar+storage+nat gas generators could be even more favorable via additional cost optimization. The desire to use industry standards that are publicly verifiable in our base case led to passing on a few opportunities for potential design optimization that are low-hanging fruit:
Alternative solar designs should be considered that sacrifice some yield (since there quite a bit of curtailment anyway) for lower cost. This could include typical fixed tilt systems, or even more uncommon approaches like PEG or Erthos. There is minimal risk in such an approach. Furthermore, this approach could be much more land-efficient as well.
This application is a good fit for DC-coupled storage, where the solar and battery storage systems sit behind shared inverters. This would result in fewer conversion losses, less solar clipping, and lower capex due to eliminating redundant and oversized power conversion. Similarly, this is a low risk opportunity.
To produce equivalent reliability to a 1.25x gas turbine design, its likely only 1x generators are needed in design cases where at least 0.5x battery storage and 0.5x solar (and often much more) is present.
Optimizing datacenter design to directly use DC power generated by solar panels was brought up by some DC operators we interviewed. This is probably difficult to achieve in practice but worth looking into.
If we introduce the above design optimizations (not including DC-native datacenters) and an estimate of their costs, the results are improved. Scenario 1 (44% renewable) becomes $87/MWh (exactly the same cost of off-grid gas turbines), Scenario 2 (82% renewable) becomes $92/MWh, and Scenario 3 (90% renewable) becomes $97/MWh.
The uptime requirements for AI training datacenters are still not entirely clear but may offer opportunities to eliminate generators entirely.
Our conversations with various stakeholders showed a range of uptime expectations. For some, the traditional datacenter requirement of five-nines was held, whereas for others (often newer players focused exclusively on model training), this requirement appeared more flexible, even down to 90%.
WIth this in mind, we considered what the LCOE would be for solar+battery only solutions that serve less than 100% of the 24/7 flat load used in the simulations and do not have redundancy to manage unplanned downtime.
The results of this model run show that for nearly the same cost as the gas turbine case, solar+storage could serve load 90% of the time (excluding unplanned downtime).
This approach would also be faster to deploy, given the gas generation components are longer lead-time than solar and battery components. In theory, one could operate this way for a period of time, and then add generators later when 100% uptime becomes necessary.
What would this look like in an unsubsidized, easy-to-permit, zero-tariffs scenario?
While not the focus of this white paper and not immediately actionable, we were curious how these costs might look in a scenario with fewer market distortions: no investment tax credit, extremely easy to build projects, no tariffs on equipment, etc. We call this our Abundance Scenario.
To test this, first we eliminated the Investment Tax Credit. Next, as a comp for “easy to build” and “no tariffs”, we took solar and battery total installed system costs (pre-subsidies) reported from China (about 50 cents/watt for solar and $145/kWh for batteries) as an aggressive low case for build costs.
We then ran those costs across various levels of redundancy. We find that, at these costs and if willing to sacrifice redundancy entirely, one could build a system that serves 95% of the 24/7 flat datacenter load with just solar and storage at a cost equal to off-grid gas turbines. And even with the 1.25x generator capacity, a 75% renewable (100% of load served) system is just $90/MWh versus $86/MWh for off-grid gas turbines.
Scale: Is there enough land to power the near-term AI race? Where?
We’ll first describe what we did and then describe the findings. What we did:
We converted the system design for these three microgrid models into land requirements. While the minimum cluster size is 500 MW, we chose 100 MW as the minimum site size based on feedback that five 100 MW sites within 10 miles of one another (a “cluster”) is roughly as good as a single 500 MW site.
Solar Capacity per Site (MW)
5 acres / MWBattery Capacity per Site (MWh)
0.01 acres / MWhGas Capacity per Site (MW)
0.05 acres / MWAcres Needed for Minimum Viable Site Acres Needed for Minimum Viable Cluster Scenario 1:
90% Renewables /
10% Gas500 1,600 100 2,525 12,625 Scenario 2:
82% Renewables /
18% Gas400 1,400 100 2,022 10,110 Scenario 3:
44% Renewables /
56% Gas200 200 100 1,008 5,040 We completed an analysis to determine how much land in the Southwest US is feasible for each system design. To start, we narrowed in on the region of the US that has the best solar resource potential as per NREL. The sample included 768.5 million acres spread across 326 counties in Texas, Oklahoma, Colorado, New Mexico, Utah, Arizona, California, and Nevada. Land within this sample region was considered feasible for development if it:
- Had a slope grade less than 15° (26.79%) as specified by the US Geological Survey
- Was at least 25 feet from wetlands and floodplains (as specified by the National Wetlands Inventory and Federal Emergency Management Agency, respectively)
- Was at least 25 feet from property boundaries, railroads, and transmission lines
If an area had enough contiguous buildable land to meet the total acreage requirement for a given scenario, as outlined in the land requirements table above, then it was considered a potential site. For example, a site consisting of at least 2,525 acres of contiguous buildable land is suitable for the 90% Renewables / 10% Gas scenario, as it assumes it can contain a 100 MW datacenter and all the requisite energy generation and storage equipment.
Once suitable land had been grouped into potential sites, Paces then filtered sites to only include those that were:4
- Within 10 miles of an interstate natural gas pipeline (to reflect proximity to the gas distribution system)
- Within 30 miles from an airport and interstate highway (to reflect ease of funneling personnel and equipment to the site during construction)
- Not on any state or federal protected lands or conservation easements (to avoid major permitting issues)
Finally, Paces further filtered the sample sites by ensuring each site was within 10 miles of enough buildable land contained on nearby sample sites to build at least 500 MW of total datacenter capacity, the minimum size for a hyperscaler “cluster” for training. This 0.5 GW of cluster capacity can consist of up to 5 sites of at least 100 MW each, or as few as 1 very large site supporting all 500 MW of datacenter capacity.
To make the type of site we’re looking for more concrete, let’s zoom in on an actual candidate site for 500 MW of datacenter capacity. The site pictured below is southwest of Odessa, Texas with the following characteristics:
12,608 acres dedicated to PV solar, spread across six solar farms
80 acres of lithium-ion batteries with 8,000 MWh of storage capacity
25 acres to host 500 MW of gas backup capacity
0.5 GW of compute infrastructure for AI training
Intersecting interstate natural gas pipeline (shown in light blue)
Clear access via nearby roads, highways
Site consists of 38 parcels of 20,200 acres total, spread across a group of 20 distinct property owners consisting of private business, holding companies, and ranchland
What we found:
There’s >1,200 GW worth of sites suitable for AI datacenter capacity in the US Southwest. In practice this means there’s plenty of buffer for things like uninterested property owners, system design constraints not accounted for in the initial screening criteria, or unexpected permitting challenges.
‘Site-suitable’ acres available Acres per MWh (per scenarios table) Total capacity in the US Southwest (GW) Scenario 1: 90% Renewables / 10% Gas 32.68M 25 1,294 Scenario 2: 82% Renewables / 18% Gas 40.55M 20 2,005 Scenario 3: 44% Renewables / 56% Gas 60.99M 10 6,051 The vast majority of sites are in West Texas. Colorado is the runner up, though far behind. This is largely due to gas pipeline density. If you relax this constraint by moving 100% off grid, you can build almost anywhere.
Most of this suitable land is privately owned, meaning most of this is commercially viable today.
As a potential bonus, ~20% of sites are eligible for an additional 10% Investment Tax Credit (ITC), which would further reduce LCOE by 1.7 cents for the 90% renewable case. The purple overlay highlights sites that fall within an Energy Employment Community or Retired Coal or Adjoining Census Tracts as outlined by the Inflation Reduction Act (if it holds).
Most eligible parcels in Paces’ data set have the contact information for the owner. Which is just to say, these aren’t just hypothetical sites, but potentially buyable land.
Speed: How fast could these be built?
- While ‘typical’ time to deployment would be ~2-4 years, there’s no obvious reason why this couldn’t be done faster by a very motivated and competent builder.
- Construction: Standard construction timelines today would be 18-24 months, but a team willing to heavily lean in and take smart risks could target ~12 months.
- Site control & permitting: Typically 12+ months but in theory nothing is stopping this from being much faster for a few reasons: (1) Site acquisition of private land is likely easier due to the massive financial returns involved for property owners and less competition from traditional energy developers. Furthermore, landowners in the US Southwest are used to entertaining land offers for energy deals—familiarity can help speed this up. (2) Permitting review varies based on state and local governance but can be very fast in states like Texas and Oklahoma with fewer environmental regulations and less restrictive zoning practices. On the other hand, solar + storage requires significantly more land procurement than other options, which leads to more negotiations with independent landowners and more surface area for permitting review.
- Off-grid solar is meaningfully faster than adding capacity to the grid via waiting for interconnection, queues for which are around 5 years in most parts of the country.
- Off-grid solar is also faster than colocated natural gas turbines (at least today) because turbine lead times are long—currently 3+ years.
- Gas turbines have an inelastic production rate and suppliers are noting high demand and limited manufacturing capacity, suggesting long lead times will continue or even increase.
- Additionally, full gas power plants have more permitting complexity around air rights which can lead to project delays.
- Gas engines have a year lead time whereas gas turbines are currently experiencing longer times of at least 3 years for the most optimistic estimates (with many considering 4-5 years more likely5).
- The one thing that could be faster is rented portable generation. This is typically a stop-gap solution due to high costs and worse reliability. Additionally, the near-term scale potential is limited.
The issue is that there’s limited supply of generators available in the rental market, and the manufacturing capacity for additional units leverages the same lines needed for permanent solutions.
As XAI discovered, these still require battery buffer to ensure sufficient power quality (i.e. frequency regulation, rapid ramping, etc.) to manage AI training load profiles. While XAI was able to quickly install batteries for their facility, not all companies would be able to rapidly procure these systems.
Long-term, rental generators will also be a significantly more expensive (>$300/MWh) and less reliable option than either dedicated gas turbines, solar + storage with dedicated gas backup, or grid connections.
Temporary, portable power, if available, can be utilized before the final project is commissioned for either solar+storage or colocated gas turbine approaches.
Climate: What would the emissions impact be?
- Avoiding emissions is not the top priority for hyperscalers. But let’s assume this off-grid solar microgrid is compelling enough to hyperscalers based on other merits—namely speed and scale—and the cost premium is tolerable.
- What would the emissions impact be if all new energy built for AI training in the next ~5 years was done with off-grid solar microgrids versus natural gas? Here we assume an emissions factor of 0.5 tons CO₂ per MWh for gas generation, and an emissions factor of 0.07 tons CO₂ per MWh for a 90% renewables / 10% gas case, accounting for lifecycle emissions. A typical 500 MW capacity datacenter would result in 2.2 million tons of CO₂ emissions per year, compared to 0.3 million tons per year for the 90% renewables option.
- The use of off-grid solar microgrids to meet all expected datacenter growth could result in substantial emissions reductions: between 0.4 billion tons (30 GW new datacenters) and 4.1 billion tons (300 GW new datacenters) of CO₂ emissions avoided between 2026 and 2030.
If this is so great, why isn’t it happening?
There seem to be a few reasons:
- To some extent, cost. There is a cost premium for a 90% renewable system ($109/MWh vs. $86/MWh). While $23/MWh is a premium, the implied cost per ton of avoided emissions here is around $50–within the range of what big tech companies pay for mitigation today. Furthermore, the 44% renewable system is basically at parity with gas only and offers a valuable hedge on fuel price risk.
- Massive datacenters dedicated to training only are a recent phenomenon, and datacenter designers have historically been skeptical of off-grid solutions due to the perceived need to optimize for uptime reliability. As more training-only datacenters are built, hyperscalers may be more willing to explore off-grid solar microgrid solutions. Interestingly, neither the off-grid gas turbine reference case nor the Three Mile Island + delivery costs case would achieve five nines without additional redundancy.
- But the biggest reason may simply be inertia and the fact that this hasn’t been done before. While building off-grid solar microgrids of this magnitude would be a first, it’s very possible to do with technology that exists today, and to scale it quickly.
Off-grid solar microgrids offer a fast path to power AI datacenters at enormous scale. The tech is mature, the suitable parcels of land in the US Southwest are known, and this solution is likely faster than most, if not all, alternatives (at least at the time of writing this paper). The advantages to whoever moves on this quickly could be substantial, and the broader opportunity offers a compelling path to rapidly securing key inputs to US AI leadership.
We hope this initial open-source exploration helps drive deeper analysis, and we welcome feedback, questions, critique, and collaboration. You can reach us at feedback@offgridai.us.